In the fast-paced world of AI hardware, the Cerebras CS-3 and Nvidia DGX B200 are two of the most exciting new offerings to hit the market in 2024. Both systems are designed to tackle large scale AI training, but they take decidedly different approaches. For ML researchers and IT buyers looking to stay on the cutting edge, understanding the strengths and trade-offs of each platform is crucial. In this in-depth comparison, we’ll put the CS-3 and DGX B200 head-to-head across the metrics that matter most for large model training, including memory capacity, compute performance, interconnect bandwidth, and power efficiency. Let’s dive in.
Cerebras CS-3 and B200 Hardware Overview
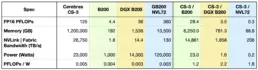
Announced at Cerebras AI Day, the CS-3 is powered by the third generation of Cerebras’ wafer scale engine – a giant wafer scale chip with 4 trillion transistors. Comprised of 900,000 AI cores connected via an on-chip fabric, the third gen wafer scale engine is not only the largest chip in production, it’s also the fastest – with 125 petaflops of AI performance. The CS-3 is connected to 12TB to 1.2PB of external memory, enabling trillion parameter models to be trained easily and efficiently. The CS-3 is a 15U server consuming up to 23kW of power. It is available today on prem or in the cloud.
Announced in GTC 2024, The Nvidia B200 “Blackwell” is the successor to the H100 GPU. The B200 is comprised of two GPU dies coupled together via NVlink with a total of 208B transistors. The B200 provides 4.4 petaflops of FP16 AI compute and comes with 192GB of memory. The B200 is available in two server formats. The DGX B200 is a 10U server with 8x B200 GPUs. It offers 36 petaflops of AI compute, 1.5TB of memory, and consumes 14.3 kW. The DGX NVL72 is a full rack solution with 72 B200 GPUs connected via NVLink. It provides 360 petaflops of AI compute and consumes 120kW of power. B200 products are expected to ship in Q4 2024.
Training Performance
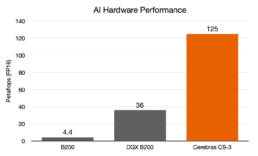
When it comes to training large AI models, the #1 determinant of performance is floating point performance. With 900,000 dedicated AI cores, the Cerebras CS-3 achieves 125 petaflops of AI compute using industry standard FP16 precision. A single Nvidia B200 GPU outputs 4.4 petaflops of AI compute while a DGX B200 with 8 GPUs totals 36 petaflops. In raw performance, a single CS-3 equates to about 3.5 DGX B200 servers, but does so in a more compact footprint, half the power consumption, and a dramatically simpler programming model.
Memory
While training performance is dictated by FLOPs, the max size of a model is dictated by the amount of memory. AI developers are constantly running into memory limitations and training frequently fails due to OOM (out of memory) errors. For example, a 13B parameter model using mixed precision, gradient accumulation, and Adam require 18 bytes of memory per parameter. This equates to 234GB of memory just for the model and optimizer states, greatly exceeding the 192GB of memory of a B200 GPU. Trillion parameter scale models only exacerbate the problem – requiring terabytes of memory, hundreds of GPUs, and complex model code to manage memory and orchestrate training.
Recognizing that LLMs require 1,000x more memory than conventional AI models, Cerebras hardware is built with a unique disaggregated memory architecture. Rather than relying on small amounts of HBM close to the GPU, we designed the dedicated, external memory device called MemoryX to store weights. MemoryX uses flash and DRAM and a custom software stack to pipeline load/store requests with minimal latency. The Cerebras CS-3 MemoryX SKUs ranging from 12 terabytes to 1.2 petabytes.
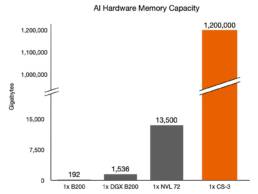
Designed for GPT-5 and beyond, our 1.2PB Hyperscale SKU trains models with 24 trillion parameters. It has 6,000x more memory capacity than a B200 GPU, over 700x more memory than a DGX B200, and over 80x the memory capacity of a full rack NVL72.
In other words, it would take 80 racks of NVL72 to match the memory capacity of a single CS-3 with MemoryX 1.2PB.
Furthermore, unlike GPU memory, MemoryX presents a single, unified memory block to the AI developer. Training a trillion parameter model is as simple as naively defining the model dimensions and starting a run. The entire model fits in memory – no refactoring is needed.
Model Size
Larger models require correspondingly larger memory capacity. Building large models on GPUs require hundreds or thousands of GPUs, making it challenging to manage and scale. The CS-3’s disaggregated memory architecture can attach petabytes of memory to a single accelerator, making it incredibly hardware efficient to work on large models.
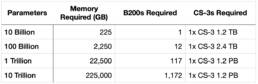
For example, a 100B parameter model requires over 2 TB of memory. Using GPU infra that would necessitate 12 B200s. The same model can be stored and trained on a single CS-3 with 2.4 TB MemoryX. A 1 trillion parameter model requires over a hundred B100s; on a single CS-3 1.2PB rack is needed to train the same model. A 10 trillion parameter model requires over 200TB of memory or over a thousands B200s – making it out of reach for most organizations. Using Cerebras hardware, a single CS-3 with 1.2 PB of MemoryX attached can load the model as simply as loading a 1B parameter model on a GPU. This makes it fast and highly capex efficient to fine-tune 100B to 10T parameter sized models.
Interconnect Bandwidth
High interconnect performance is critical to achieving high utilization across multiple chips during training. In GPU servers such as the DGX B200, this is achieved via NVLink, a proprietary interconnect that provides a dedicated link between the 8 GPUs inside the server. Nvidia’s fifth gen NVlink fabric provides 14.4TB/s of aggregate bandwidth across 8 GPUs – an impressive figure compared to conventional processors.
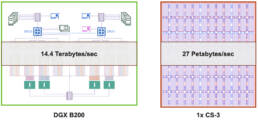
The Cerebras CS-3 interconnect system is built using an entirely different technology. Instead of using external interconnects between cores, we use on-wafer wiring to connect hundreds of thousands of cores together, providing the highest performance fabric at the lowest power.
The CS-3 on-wafer fabric provides 27 petabytes per second of aggregate bandwidth across 900,000 cores. By comparison, this is more bandwidth than 1,800 DGX B200 servers. Even when compared to the full rack, 72 GPU NVL72, a single CS-3 provides more than 200x the interconnect bandwidth. By keeping the bulk of compute and IO on-wafer, we obviate the need for exotic interconnects, power hungry wiring, and complex programming models.
Power
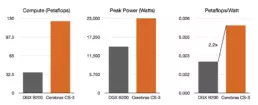
Besides raw performance, data center operators greatly value efficient power usage since it directly affects total cost of ownership. AI hardware with its tremendous compute density is especially power hungry. The CS-3 consumes 23kW peak while the DGX B200 consumes 14.3 kW. However, the CS-3 is significantly faster, providing 125 petaflops vs. 36 petaflops of the DGX B200. This translates to a 2.2x improvement in performance per watt, resulting in more than halved power expenses over the system’s operational lifespan.
Final Words
The Cerebras CS-3 and Nvidia DGX B200 are two compelling platforms for ML teams tackling large scale AI. While both systems deliver impressive speedups, the CS-3 is a compelling choice for ML engineers and organizations looking to efficiently train large AI models that can transform their businesses in record time. With its ability to eliminate the complexity of distributed training, deliver superior performance per watt, and enable unprecedented scalability, the CS-3 is poised to become the platform of choice for those pushing the boundaries of AI innovation. CS-3, unlike B200, is ready NOW for your AI needs. Contact us today if you’d like to go faster and bigger than you ever imagined.
Related Posts
May 17, 2024
Cerebras Breaks Exascale Record for Molecular Dynamics Simulations
Cerebras has set a new record for molecular dynamics simulation speed that goes…
May 1, 2024
Supercharge your HPC Research with the Cerebras SDK
Cerebras SDK 1.1.0, our second publicly available release, includes initial…